Hugues Van Assel
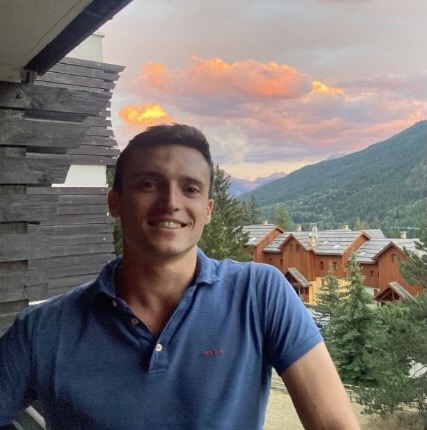
My primary research interests are in unsupervised and self-supervised learning. I develop computational methods that leverage optimal transport and probabilistic modeling to compute robust and grounded data representations suitable for real-world applications. I am especially interested in applying these methods to tackle challenges in cell biology.
I am a strong advocate for open and accessible science through projects such as:
- TorchDR : a modular, GPU-friendly toolbox for dimensionality reduction (DR) that offers a unified interface for state-of-the-art DR methods.
I am a PhD student in the department of Mathematics (UMPA) of Ecole Normale Supérieure de Lyon with Aurélien Garivier and Titouan Vayer. I am currently a visiting research fellow at Brown University in the CS department with Randall Balestriero. Before my PhD, I was a student at Ecole polytechnique and MVA master (CV).
news
Sep 17, 2024 | TorchDR 0.1 is out ! Feel free to give it a try ! |
---|---|
Feb 12, 2024 | New preprint out! Exciting work relating dimensionality reduction and clustering methods to the Gromov-Wasserstein OT problem. |
Dec 10, 2023 | I will be at NeurIPS 2023 to present SNEkhorn as well as two posters at the OTML workshop. |
Jun 30, 2023 | Had an exciting week at the Generative Modelling Summer School. Thanks a lot to the organizers! |
selected publications
- PreprintDistributional Reduction: Unifying Dimensionality Reduction and Clustering with Gromov-WassersteinPreprint, 2024